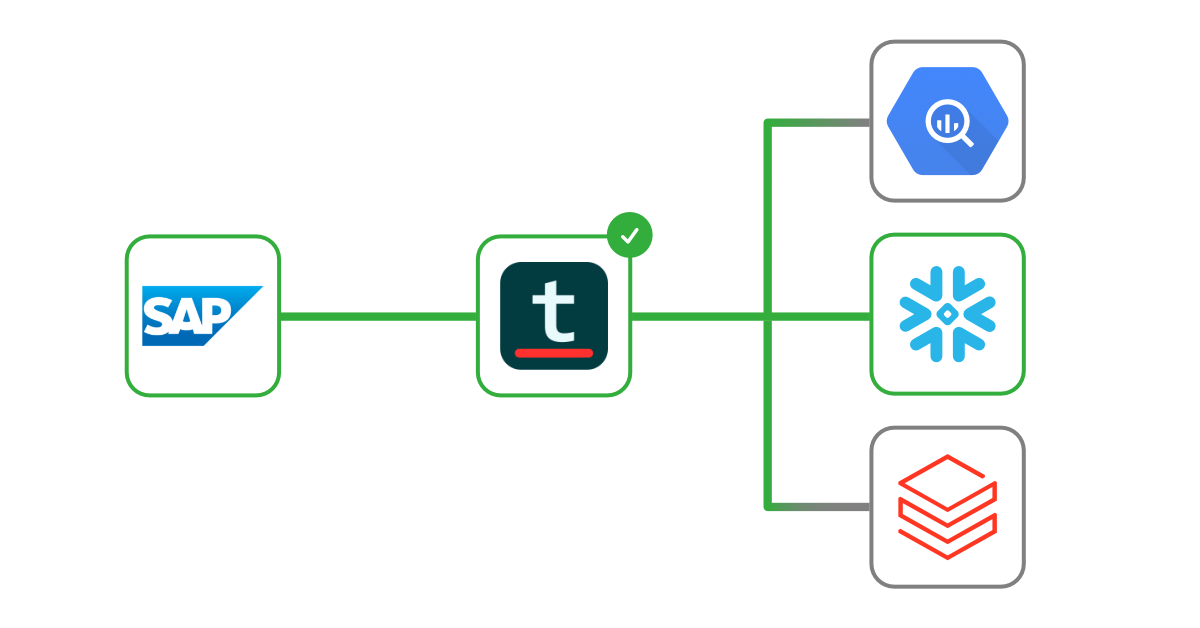
Why Data Validation Matters: The Hidden Cost of Bad Data
Every day, businesses make thousands of decisions based on replicated data. But here’s the uncomfortable truth: most organizations don’t know if their data is accurate.
This is especially critical for enterprises relying on SAP as their primary source of truth. SAP systems store vital operational data – from finance and supply chain to human resources and customer transactions – yet replication of SAP data is notoriously difficult.
The Costly Assumption
We assume our data replication processes work perfectly. We trust that when data moves from one system to another, it arrives complete and unchanged. This assumption costs businesses millions in:
- Incorrect business decisions
- Wasted engineer time
- Delayed projects
For SAP-driven organizations, this risk multiplies as complex ERP data structures with thousands of tables flow through various transformation layers before reaching decision-makers. Each transformation step introduces potential points of failure that can compromise data integrity.
Why Traditional Solutions Fall Short
The standard approach to data validation is fundamentally flawed:
- Manual Sampling: Checking a few records and hoping they represent the whole
- Basic Row Counts: Assuming matching totals mean matching data
- Reliance on end user testing: This takes valuable time better spend elsewhere for partial results
These methods might have worked when data volumes were smaller and systems simpler. Today they don’t scale, require a lot of time and effort to perform, often ignore how changes are applied to the data platform and still don’t provide complete and reliable information
The Real Problem
Data validation isn’t just a technical challenge – it’s a business risk. When teams can’t trust their data:
- Decisions get delayed while data is manually verified
- End users frequently complain that dhe data just “doesn’t feel right”
- Engineers spend countless hours investigating discrepancies, reacting to requests
- Business users create redundant checks and balances
- Everyone works slower because no one trusts the numbers
This creates a vicious cycle where technical teams are not in the driver seat and instead become overwhelmed with validation requests, while business users grow increasingly skeptical of the data they’re provided.
A Better Way Forward
We built Tracelake specificaly to adress this problem for SAP customers.
Because we believe data validation should be:
- Automatic: No more manual sampling
- Complete: Every record checked, not just a sample
- Efficient: Quick results, without bloating database costs
- Periodic: Data should be continously monitored
- Actionable: Clear insights about what’s wrong
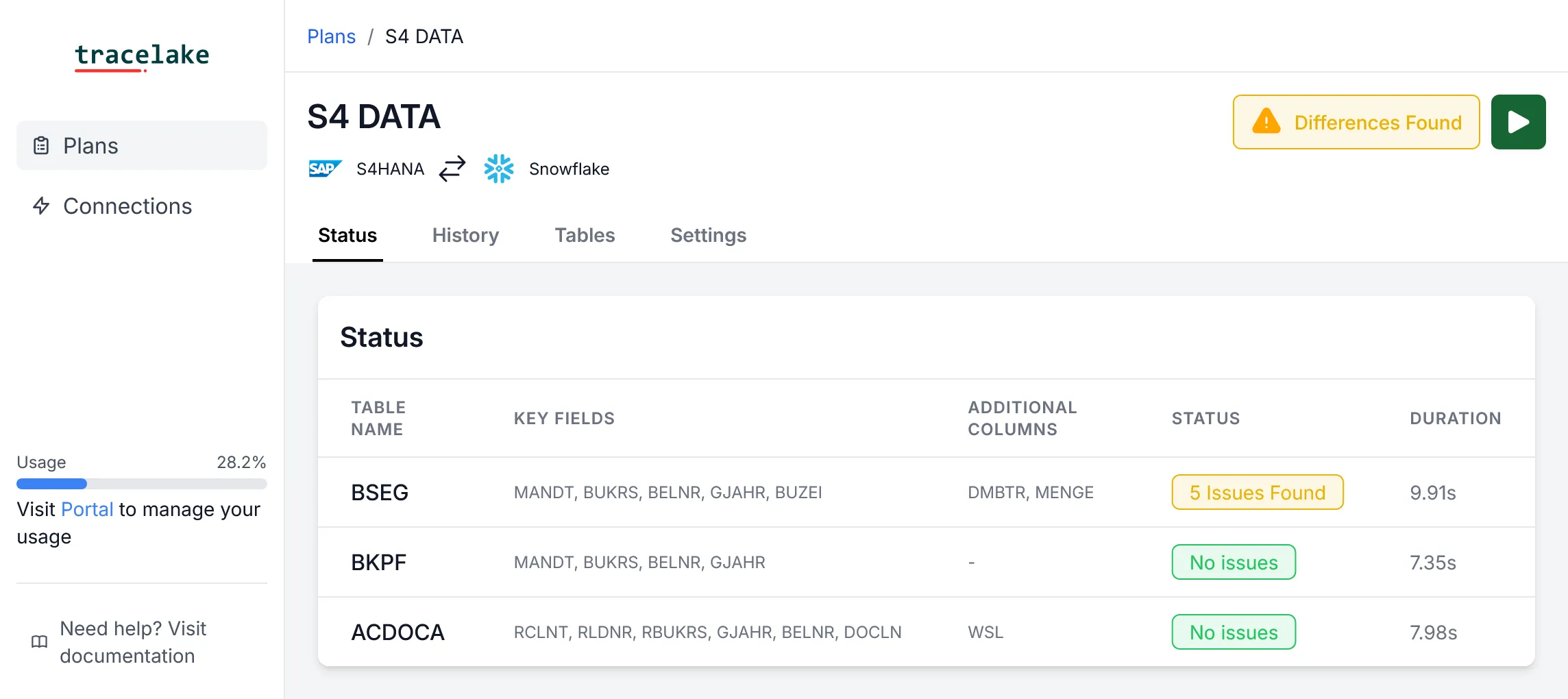
Time for Change
With the increasing reliance on real-time data for critical business decisions, comprehensive validation isn’t a luxury – it’s a necessity. Organizations with SAP at their core deserve confidence that their valuable source data remains pristine as it moves through their analytics ecosystem.
It’s time we stopped hoping our data is correct and started knowing it is.
Ready to take control of your data validation? Let’s talk.